Utilizing the power of AI & Gen AI to discover patterns and information in CMS public datasets.
Introduction
The era of data-driven decision-making, the healthcare industry stands as one of the most prominent beneficiaries of advanced technologies. The Centers for Medicare and Medicaid Services (CMS) maintains vast repositories of healthcare data that are instrumental in guiding policies, enhancing patient care, and fostering innovation[^1]. However, the sheer volume and complexity of these datasets can be overwhelming for traditional analytical methods. This is where the power of Artificial Intelligence (AI) and Generative AI (Gen AI) comes into play. In this blog, we will explore how these technologies are transforming our ability to discover patterns and unlock valuable information within CMS public datasets, and how a platform like CloudAstra can match these capabilities.
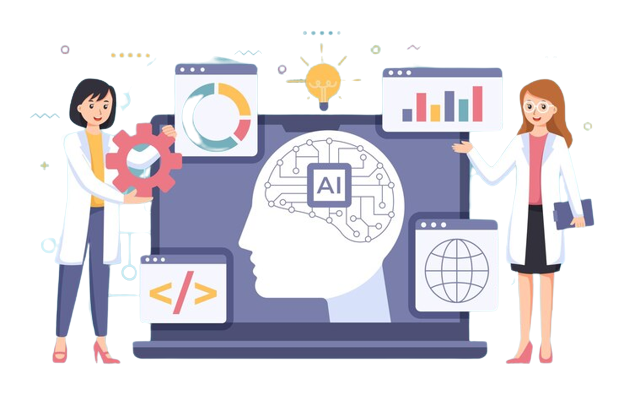
The CMS Datasets: A Treasure Trove of Healthcare Information
CMS administers several public datasets, including Medicare claims, Medicaid data, and data on the Affordable Care Act[^2]. These datasets contain a wealth of information, encompassing healthcare utilization, cost quality, and demographic details of millions of patients across the United States. Analyzing this data can lead to insights that shape the future of healthcare delivery and policy.
Key Features:
Vast Data Storage: CMS datasets are vast and require robust data storage capabilities. [CloudAstra](https://www.cloudastra.ai) offers
scalable cloud storage solutions that can accommodate the growing volume of healthcare data.
Example Datasets:
- Medicare Claims Data: This dataset includes information on healthcare claims submitted to Medicare, offering insights into diagnoses, treatments, and costs.
- Medicaid Data: Medicaid datasets provide information on health services provided to low-income individuals, offering a view into the healthcare disparities that AI can help address.
- Affordable Care Act Data: These datasets include information on insurance coverage, subsidies, and demographic data of individuals enrolled under the ACA.
The Challenge: Big Data Complexity
The primary challenge in harnessing CMS public datasets lies in their scale and complexity. The sheer volume of records and the interconnected nature of healthcare data make it a daunting task for traditional analytics. Human analysts would require immense time and resources to uncover meaningful patterns, which is where AI and Gen AI come to the rescue.
Key Features:
- Big Data Processing: [CloudAstra](https://www.cloudastra.ai) is equipped with distributed computing capabilities, allowing it to process large healthcare datasets in parallel, significantly reducing analysis time.
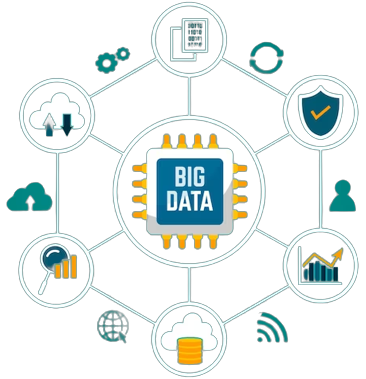
AI: Enabling Data-Driven Insights
AI, powered by machine learning and deep learning techniques, has revolutionized the way we extract insights from healthcare data. Here are some ways AI assists in this endeavor:
Example AI Applications:
- Data Preprocessing: AI can automate the process of cleaning and structuring data, which is often messy and unorganized in its raw form. It identifies and corrects errors, missing values, and inconsistencies[^3].
- Predictive Analytics: Machine learning models can predict disease trends, patient outcomes, and healthcare costs. By analyzing historical data, AI identifies patterns that humans might overlook[^4].
- Anomaly Detection: AI can swiftly identify anomalies in healthcare data. These anomalies could be fraudulent claims, unusual healthcare patterns, or potential issues with the data itself[^3].
- Natural Language Processing (NLP): NLP models can extract valuable information from unstructured text data, such as clinical notes and patient reviews, enabling a more comprehensive analysis[^3].
- Image Recognition: For datasets that include medical images, AI can assist in diagnosing conditions, tracking disease progression, and evaluating treatment outcomes[^3].
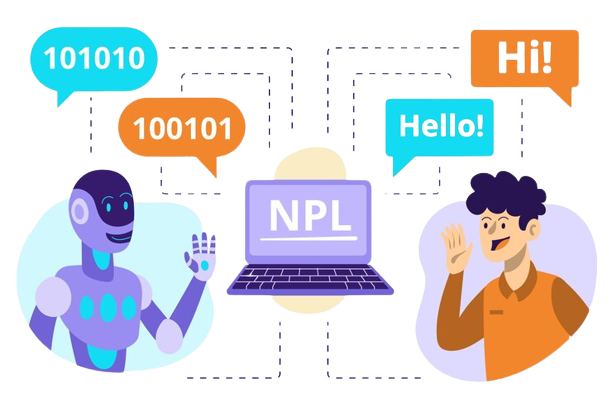
Key Features:
AI Integration: CloudAstra seamlessly integrates AI models for data preprocessing, predictive analytics, anomaly detection, NLP, and image recognition, empowering healthcare professionals with AI-driven insights.
Gen AI: Beyond Analysis to Creation
Generative AI, or Gen AI, takes the capabilities of AI a step further. It can create content, generate hypotheses, and design experiments. In the context of CMS datasets, Gen AI:
Example Gen AI Applications:
- Generates Insights: Gen AI can propose hypotheses, create data visualizations, and suggest new research directions based on the patterns it identifies in the data[^5].
- Content Creation: It can produce reports, summaries, and even research papers, simplifying the dissemination of findings and making the information more accessible to a wider audience[^5].
- Personalized Healthcare: Gen AI can create personalized care plans based on a patient’s medical history, genetics, and preferences, improving the quality of care[^5].
Key Features:
Gen AI Integration: [CloudAstra](https://www.cloudastra.ai) provides Gen AI capabilities, assisting in hypothesis generation, content creation, and personalized healthcare recommendations.
Challenges and Ethical Considerations
While AI and Gen AI hold immense potential in unlocking insights from CMS datasets, there are important challenges and ethical considerations to address:
- Data Privacy: Safeguarding patient data privacy paramount, necessitating robust security and anonymization measures.
- Bias Mitigation: Ensuring fairness in AI models monitoring and mitigating biases inherited from training data.
- Regulatory Compliance: Adherence to healthcare regulations is crucial to ensure the safe and ethical use of AI in healthcare.
- Transparency: Providing transparency in AI algorithms and decision-making processes for trust and accountability.
- Informed Consent: Ethical considerations include obtaining informed consent for data usage and maintaining transparency in AI-assisted healthcare decisions.
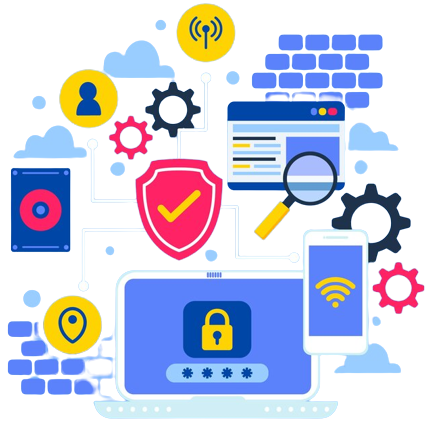
References:
- [Obermeyer, Z., & Emanuel, E. J. (2016). Predicting the Future—Big Data, Machine Learning, and Clinical Medicine. New England Journal of Medicine, 375(13), 1216-1219](https://www.nejm.org/doi/full/10.1056/NEJMp1606181).
- [Chen, I. Y., & Szolovits, P. (2013). Health care and big data. Journal of Law, Medicine & Ethics, 41(1), 56-61](https://journals.sagepub.com/doi/10.1111/jlme.12049).
- Privacy: Ensuring patient data privacy is of utmost importance. Anonymization and robust security measures are necessary to prevent data breaches[^2].
- Bias: AI models can inherit biases from the data they are trained on. Careful monitoring and bias mitigation strategies are required to ensure fairness in healthcare decisions[^1].
- Regulation: The healthcare industry is highly regulated. AI applications must comply with existing regulations to ensure safe and effective use[^1].
Conclusion
The utilization of AI and Gen AI in the analysis of CMS public datasets is a powerful tool that can transform healthcare. By automating data analysis, predicting healthcare trends, and generating insights, these technologies can enhance the quality of care, reduce costs, and drive policy improvements. A platform like [CloudAstra](https://www.cloudastra.ai), designed to match the key features needed for CMS data analysis, plays a pivotal role in harnessing the potential of AI and Gen AI. However, they must be used responsibly, with privacy and ethics at the forefront of the implementation. As we continue to explore the potential of AI and Gen AI, the future of healthcare promises to be data-driven, efficient, and patient-centric.
Contact Us
Let us innovate together. If you are interested in exploring this further contact us at https://cloudastra.ai/contact-us