Optimizing Healthcare Operations: Harnessing the Potential of Language Models
Optimizing Healthcare Operations: Harnessing the Potential of Language Models
Introduction:
The constant evolution of healthcare brings with it a massive influx of documentation, ranging from patient records to administrative paperwork, which often leads to burnout and system inefficiencies. Recent breakthroughs in domain-specific language models, particularly within healthcare, demonstrate the potential to streamline document processing and alleviate the burden on healthcare professionals. Within this blog post, we’ll examine an intriguing case study that we conducted involving the experimentation of various LLMs for healthcare entities & relations recognition and analysis.
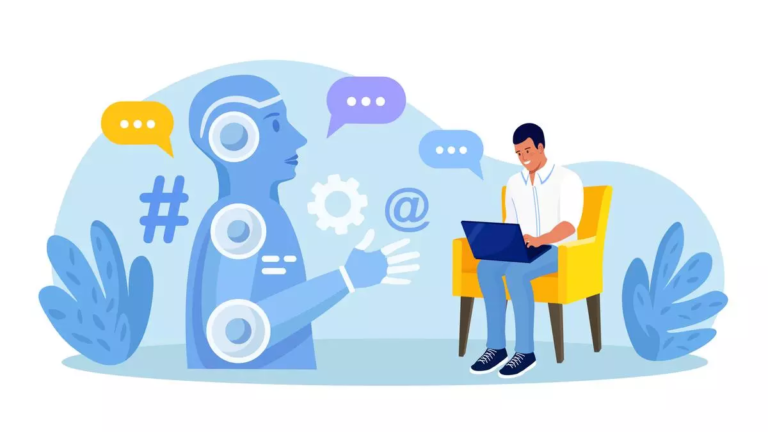
Understanding the Case: Leveraging Healthcare LLMs for Document Processing
In the healthcare industry, document processing involves extracting valuable information from diverse sources, such as medical records, diagnostic reports, and patient notes. Typically, this process involves manual tasks and consumes substantial time, resulting in a significant workload for nursing staff and healthcare providers. By leveraging the capabilities of healthcare LLMs, organizations can automate and optimize this process, thereby saving precious time and resources.
Exploring the LLMs: A Comparative Analysis
AWS Health Data Lake:
Our team has successfully streamlined document processing and extracted valuable insights from healthcare data using AWS Health Data Lake. By leveraging features like querying and entity recognition, we’ve enabled real-time analysis of patient records and diagnostic reports, leading to informed decision-making and improved patient care outcomes. AWS Health Data Lake offers a comprehensive set of tools for processing and analyzing healthcare data, including features like querying, entity recognition, and data transformation, all operating on a usage- based pricing model. With its low latency, AWS Health Data Lake is ideal for real- time document processing requirements. However, some organizations may encounter challenges due to the learning curve associated with AWS services and potential costs. Nonetheless, AWS Health Data Lake remains a valuable option for healthcare document processing, especially with its integration with other AWS services and extensive documentation.
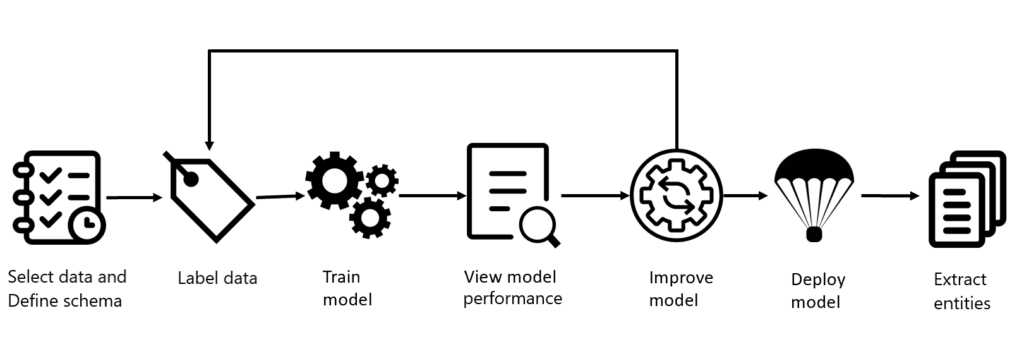
Azure Text Analytics:
Utilizing the text analytics LLM tailored for healthcare, we capitalized on its named entity recognition (NER), relation extraction, and summarization capabilities to derive precise insights from healthcare data. This enabled us to extract healthcare-related entities and their relations, including treatments, diagnoses, ICD codes, and SNOMED codes, ensuring comprehensive analysis. Additionally, we successfully summarized the content within healthcare reports with accuracy. The pricing model based on inference per 1,000 tokens, and the LLM’s accessibility as a cloud-supported API, facilitated swift document processing. Moreover, accessing the Azure AI Language resource was straightforward, ensuring smooth integration and enhancing our workflow. With its seamless integration with other Azure services, competitive pricing, comprehensive features, and user-friendly interface, it stands as a compelling option for healthcare document processing. Notably, the analysis conducted using the Text Analytics LLM was comparable to the AWS counterpart, with the key distinction that the AWS solution lacks summarization capabilities.
Google Healthcare Natural Language API:
We utilized the Google Healthcare Natural Language API to process healthcare data and identify medical entities, their relations, and other insights. While this API excelled in entity recognition and relation extraction, it lacked additional features required for comprehensive analysis. If the primary objective of the application is healthcare entity extraction, this API is a viable option; however, for more comprehensive analysis, other AI-language models from Google could be considered. Despite its limitations, the Google Healthcare Natural Language API offers a straightforward interface and seamless integration, minimizing the need for extensive engineering efforts. Leveraging this API streamlines the process of healthcare data processing and entity identification, making it an efficient solution for targeted tasks.
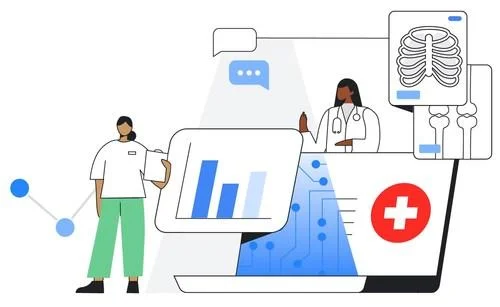
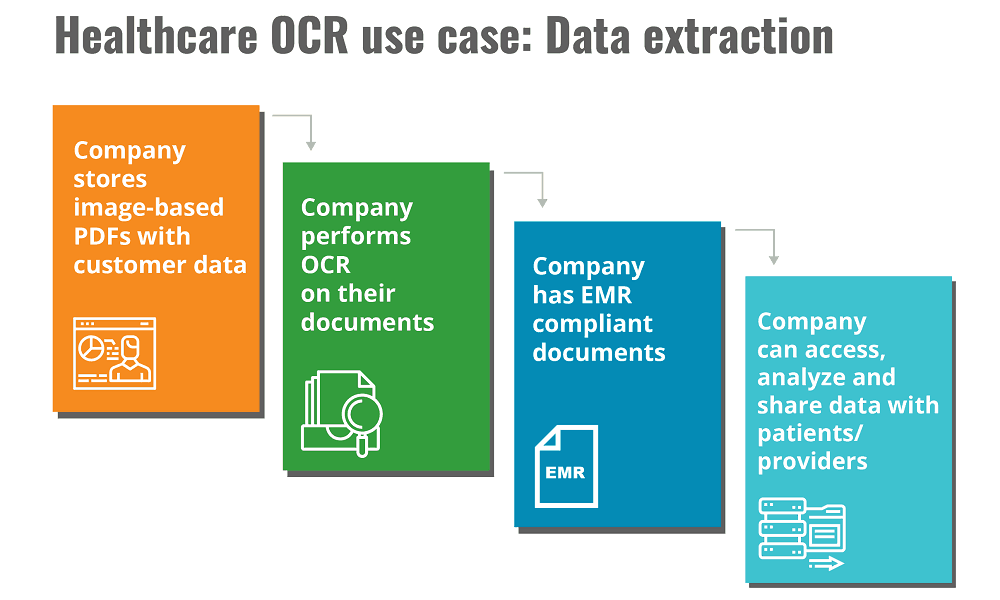
Google MedPalm2:
Google MedPalm2 distinguishes itself as an advanced healthcare LLM notable for its exceptional capability to recognize complex medical entities and relations, alongside its support for medical Q&A, thus differentiating it from other LLMs. Nevertheless, it’s important to acknowledge that accessing Google MedPalm2 is restricted and requires requesting access from the Google team, along with providing detailed information about the intended usage. This procedural requirement may present challenges for organizations aiming to explore the service, and as a result, we are yet to experiment with this model. Despite this initial hurdle, Google MedPalm2 boasts both low latency and advanced capabilities, rendering it an appealing choice for organizations seeking cutting- edge solutions in healthcare document processing.
Conclusion:
To conclude, utilization of healthcare LLMs for document processing signifies a paradigm shift in healthcare operations. This case study on prominent LLMs including AWS Health Data Lake, Azure Text Analytics, Google Healthcare Natural Language API, and Google MedPalm2 for healthcare data processing and efficient medical entity recognition underscores their profound impact on operational efficiency, staff well-being, and patient care outcomes. While each LLM offers unique advantages and considerations, their collective impact on healthcare document processing is undeniably profound. As technology continues to evolve, embracing LLMs will be crucial in shaping the future of healthcare delivery.
Contact Us:
Let us innovate together. If you are interested in exploring this further contact us at https://cloudastra.ai/contact-us