Ensuring Ethical Excellence: A Guide to Responsible AI Implementation
Ensuring Ethical Excellence: A Guide to Responsible AI Implementation
Introduction:
Discover the innovation frontier in AI application! Uncover the advantages of non-clinical use cases—where verifiable decisions and non-PHI data create the perfect ground for groundbreaking advancements, contrasting with clinical settings. Explore the potential and ethical implications of AI beyond healthcare in this insightful blog.
Clinical and non-clinical use cases:
Clinical: Medical settings using technology for diagnosis, treatment, and patient care. Non-clinical: Applications outside medical environments like wellness, fitness, and research.
Requirements for ethical use of AI:
- Explainability: AI must be transparent in its decision-making to build trust and accountability.
- Predictability Testing: Rigorous testing ensures AI reliability and consistent outcomes.
- AI as Healthcare Co-pilot: It should support healthcare workers, enhancing diagnostics and patient care.
- Collaborative Partnership: Emphasize a symbiotic relationship between humans and AI for effective problem-solving and innovation.
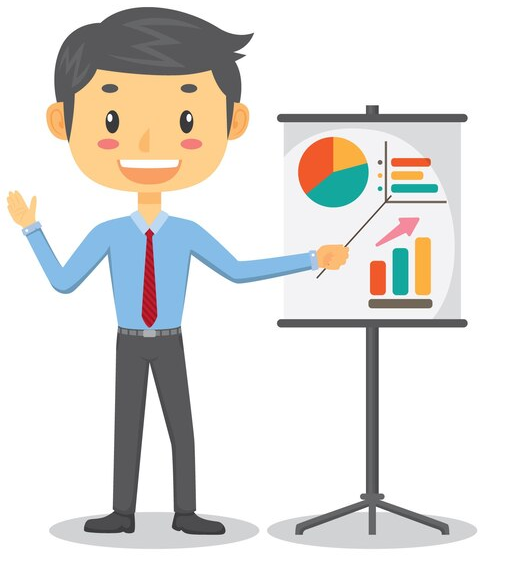
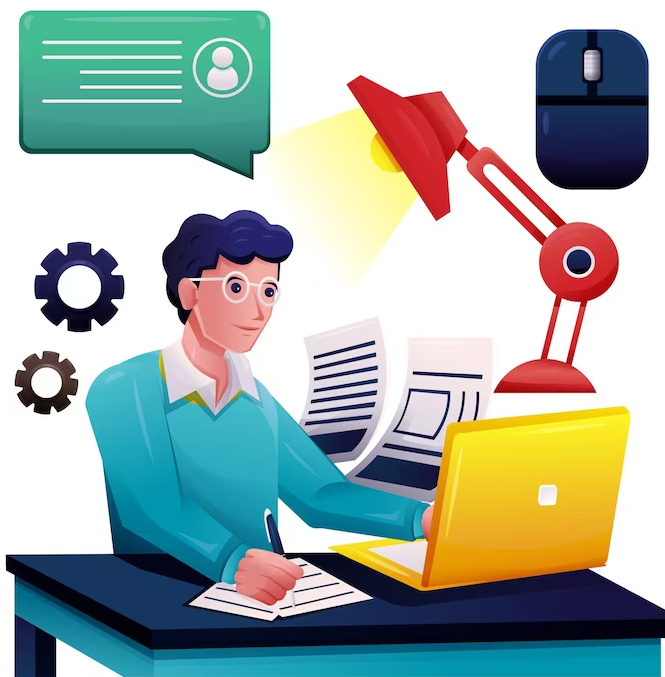
Risks of using AI:
- Verifiable AI Recommendations: Ensure AI suggestions are verifiable and explainable to maintain trust and accuracy.
- Manual Override Capability: Always have a manual override to rectify or counter incorrect AI decisions, preventing over-reliance on automated processes.
- Avoiding Human Overextension: AI shouldn’t be used to exploit or push individuals beyond reasonable limits, as seen in instances like ride-share apps pressuring drivers, potentially impacting well-being.
Gen AI:
- Risk in Gen AI Data: Data sent to Gen AI carries inherent risks, regardless of content, demanding careful handling and privacy measures.
- Preference for Non-PHI Operational Data: Utilizing operational data devoid of Personal Health Information (PHI) reduces associated risks and enhances data safety.
Why non-clinical use cases are a sweet spot for innovation:
- Non-PHI Data Dominance: Non-clinical applications predominantly involve non-Personal Health Information (PHI), reducing the complexity of data handling and privacy concerns.
- Verifiability in Decision-Making: Decisions in non-clinical scenarios, such as predicting wait times, are easier to verify compared to critical clinical decisions like image classification for diseases, making innovation and validation more straightforward.
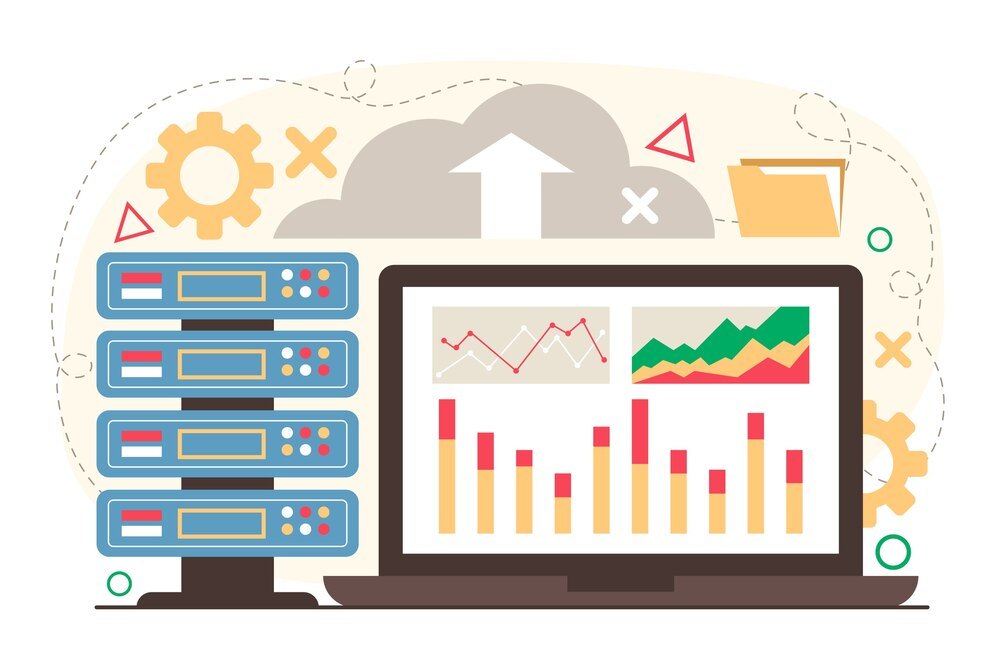
Conclusion:

Contact Us
Let us innovate together. If you are interested in exploring this further contact us at https://cloudastra.ai/contact-us